Metrics, Rankings, and Partnership for an Incentive-Based Budget
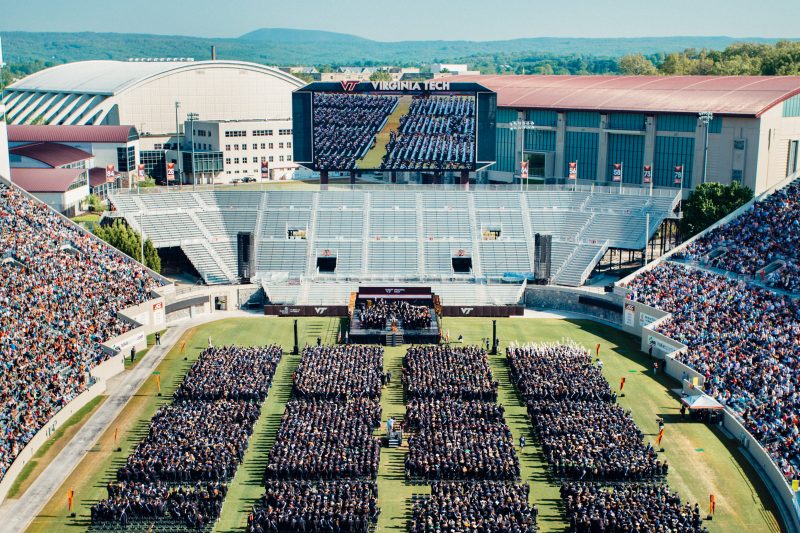
Informing Multi-Year Goals
White papers on the design and use of metrics, rankings, and the Partnership for an Incentive-Based Budget (PIBB) model were developed to inform multi-year goals.

METRICS WHITE PAPER
ON THE DESIGN AND USE OF METRICS
By the Strategic Planning Metrics Sub-Committee - June 22, 2018
The purpose of this document is two-fold. First, it serves as a guide for the Strategic Planning Committee as it drafts the 2019-2025 Virginia Tech Strategic Plan, particularly in terms of the metrics the Committee will choose to assess progress towards the strategic objectives. Second, it provides some guidelines for the larger Virginia Tech community, particularly administrators responsible for defining and implementing metrics throughout other parts of the academic enterprise, on how to design and use metrics.
In writing this, we take the point of view that in any large organization key metrics are indispensable for understanding and communicating organizational performance: They help report progress and guide decision making. Furthermore, we recognize that some metrics will be used, whether Virginia Tech likes it or not, by external organizations in such things as university rankings. Given the impact of these rankings on the university, it is thus critical that such metrics are not ignored and, in fact, perhaps actively managed.
On the other hand, we are also cognizant that there is a proliferation of metrics throughout society that follows from a frequently misplaced faith that metrics: (1) can be used to fully characterize an individual’s or organization’s performance, and (2) that they are useful for properly and positively incentivizing behavior. As Wilsdon et al. (2015) say in their report, The Metric Tide: Report of the Independent Review of the Role ofMetrics in Research Assessment and Management,
Metrics evoke a mixed reaction from the research community. A commitment to using data and evidence to inform decisions makes many of us sympathetic, even enthusiastic, about the prospect of granular, real-time analysis of our own activities. If we as a sector can’t take full advantage of the possibilities of big data, then who can?
Yet we only have to look around us, at the blunt use of metrics such as journal impact factors, h-indices and grant income targets to be reminded of the pitfalls. Some of the most precious qualities of academic culture resist simple quantification, and individual indicators can struggle to do justice to the richness and plurality of our research. Too often, poorly designed evaluation criteria are “dominating minds, distorting behaviour and determining careers.” At their worst, metrics can contribute to what Rowan Williams, the former Archbishop of Canterbury, calls a “new barbarity” in our universities. The tragic case of Stefan Grimm, whose suicide in September 2014 led Imperial College to launch a review of its use of performance metrics, is a jolting reminder that what's at stake in these debates is more than just the design of effective management systems. Metrics hold real power: they are constitutive of values, identities and livelihoods.
As this paper should make clear, it is critical to carefully select and define metrics, as well as ensure the quality of the data upon which the metrics are calculated. It is equally critical that consumers of the metrics have a nuanced understanding of what each metric does and does not measure as well as how a metric may incentivize behavior, potentially with both intended and unintended consequences.
EXECUTIVE SUMMARY
In the following, we explain what constitutes a good metric. Several factors are paramount: ease of measurement, direct correlation to institutional success, predictive of good performance, control by the group being measured, and comparableness to competitors' measures. We also identify key characteristics of quality data: relevance, accuracy, timeliness, accessibility, interpretability, coherence, and credibility. Finally, we foreground key principles for developing metrics. These encompass careful definition, paucity in number, reliable data, isomorphic comparison, cost sensitivity, meaningful ratio expression, minimization of perverse incentives, distinction between target and measurement, and care to ensure ease of measurement does not determine target of measure.
In this section, we provide a few key definitions, including defining the term “metric,” then we discuss the characteristics of a good metric, and finally we distinguish between “direct” and “proxy” metrics/measures.
To begin, the use of the word “metric” in the context of strategic planning or organizational management is somewhat more specific than the typical dictionary definition. For example, Merriam-Webster (2018) defines a metric either as “a standard of measurement” or in terms of its formal use in mathematics. The Oxford Living Dictionary (2018) comes closer to our usage amplifying the main definition of “A system or standard of measurement” with “(in business) a set of figures or statistics that measure results.” For our purposes, we use
METRIC: A quantifiable measure used to track or assess an individual’s, organization’s, or process’s progress towards a specific objective.
Citation-based metrics are often referred to as bibliometrics, and the term altmetrics refers to alternative metrics that focus on trying to measure the impact of research in alternative forums such as social media. Metrics can be direct or proxy measures of progress towards an objective. A direct measure is one that, as the name suggests, is based on data that directly measure the objective. For example, for an objective focused on achieving a particular enrollment target, a direct metric is the number of students enrolled in the university at, say, the start of the fall semester. On the other hand, a proxy measure is one that is based on data that only indirectly measure the objective. For example, SPOT scores directly measure student perceptions of teaching but are intended to be proxy measures of actual teaching performance.
Metrics can be used to assess performance and communicate preferences or as a way to influence organizational behavior. As is discussed in more detail below, designing metrics to influence behavior is the more difficult of the two, both because the measurement becomes less reliable over time as behavior adapts and because it can have unintended consequences potentially leading to unforeseen outcomes.
DEFINING A GOOD METRIC
Not all metrics are good in the sense that they can be ill-defined and/or ill-applied in any number of ways. See, for example, Muller (2018a). And, while it is impossible to catalog all the ways that metrics can be misapplied and misused, there are some guidelines about what makes a good metric.
We begin by paraphrasing the five characteristics of a good metric by Trammell (2016):
A good metric should be relatively simple to measure. If you have to build a new system or implement a complicated process just to measure the metric, it's probably not worth measuring in the first place.
The metric should be tied to institution-oriented goals you establish for the department, group, or company. The right metric will tell you if you are successfully executing the fundamentals.
The best metrics do not tell you just how well you've done (for a business, financials provide that measure); they tell you how well you're going to do - in the next month, semester, or year.
It's difficult to do, but identifying those fundamentals pertaining to a particular team will tell you much more about their strengths and performance.
It's helpful to track your progress against peer institutions. This will help judge how well you're building or maintaining an operational advantage, holding on to top talent, and retaining students.
Inherent in these characteristics is the quality of the data, since easily measured poor data is still just poor data, and that to have comparable metrics one must also have data on one's competitors that is directly comparable. As Godfrey (2008) said,
"Data quality is a critically important subject. Unfortunately, it is one of the least understood subjects in quality management and, far too often, is simply ignored."
We return to the question data quality in the next section.
Building on these, Yoskovitz (2013) says a good metric is:
"Being able to compare a metric across time periods, groups of users, or competitors helps you understand which way things are moving."
"Take the numbers you're tracking now...if people can't remember the numbers you're focused on and discuss them effectively, it becomes much harder to turn a change in the data into a change in the culture."
"Ratios and rates are inherently comparative. For example, if you compare a daily metric to the same metric over a month, you'll see whether you're looking at a sudden spike or a long-term trend."
"This is by far the most important criterion for a metric: what will you do differently based on changes in the number? If you don't know, it's a bad metric."
Of course, the assumption in the last characteristic above is that the metric changes behavior in a positive way (which could also mean reinforcing current behavior) and not a negative one. In choosing metrics, it is critical to assess this, and particularly the issue of whether the metric could drive unintended changes in behavior (perverse incentives). See Muller (2018a) for examples of unintended consequences and Edwards and Roy (2016) for illustrations of how well intended metrics can result in perverse incentives. We return to this point later.
The TWDI Blog (2010) lists twelve characteristics of effective metrics. Some of these are redundant with previous characteristics, so here we list the unique ones:
"To create effective performance metrics, you must start at the end point-with the goals, objectives or outcomes you want to achieve-and then work backwards. A good performance metric embodies a strategic objective."
"Actionable metrics require timely data. Performances metrics must be updated frequently enough so the accountable individual or team can intervene to improve performance..."
"For users to trust a performace mentric, they must understand its origins. This means every metric should give users the option to view its metadata, including the name of the owner, the time the metric was last updated, how it was calculated, systems of origin, and so on."
"It is difficult to create performance metrics that accurately measure an activity. Part of this stems from the underlying data, which often needs to be scanned for defects, standardized, and deduped, and integrated before displaying to users. Poor systems data creates lousy performances metrics that users won't trust. Garbage in, garbage out."
"Performance metrics are designed to drive desired outcomes. Many organizations create performance metrics but never calculate the degree to which they influence the behaviors or outcomes they want."
"A performance metric has a natural life cycle." When first introduced, the performance metric energizes the institution and performance improves. Over time, the metric loses its impact and must be refreshed, revised, or discarded.
Some of these characteristics have to do with the quality of the data, including the TIMELY, REFERENCEABLE, and ACCURATE characteristics, and we will delve into the question of defining data quality more in the next section. The CORRELATED characteristic makes the point that metrics intended to influence behaviors should influence the desired behaviors and the RELEVANT characteristic connects back to the notion of continuous planning.
A good metric is one that is well defined, quantifiably measurable, and if we model it in the form of a “key result” as described by Doerr (2018), it has a numeric goal. As described in Doerr (2018, p. 23), Andy Grove, the former CEO of Intel, described his system of objectives and key results as follows:
Now the two key phrases...are objectives and the key result. And they match the two purposes. The objective is the direction: “We want to dominate the mid-range microcomputer component business.” That’s an objective. That’s where we’re going to go. Key results for this quarter: “Win ten new designed for the 8085” is one key result. It’s a milestone. The two are not the same…
The key result has to be measurable. But at the end you can look, and without any arguments: Did I do that or did I not do it? Yes? No? Simple. No judgements in it.
Now, did we dominate the mid-range microcomputer business? That’s for us to argue in the years to come, but over the next quarter we’ll know whether we’ve won ten new designs or not.
What is interesting in this approach is the combination of a numeric goal with the metric itself to form a “key result.” In so doing, this unburdens the objective from having to have a numeric goal and so it can simply express the desired organizational direction. Such a system has the potential to help the strategic plan align better with the notion of continual planning where, say, the strategic plan can specify six-year objectives andperhaps key results but the key results (and thus intermediate goals) can be updated more frequently.
In the context of a university, the Educational Advisory Board (EAB) defined the following seven metrics characteristics in their report “Academic Vital Signs: Aligning Departmental Evaluation with Institutional Priorities.” Their intention is to ensure that “[b]road institutional metrics [can be] translated into clear, actionable goals for academic departments in order to motivate improvement” (EAB, 2018, p. 8):
- ALIGNED: Do department-level changes in the metric reflect the relevant institutional goal(s)?
- MEASURABLE: Can the institution collect longitudinal information about the metric?
- REALISTIC/FAIR: Does the metric control for variables outside departmental influence?
- ACTIONABLE: Does the department have direct influence over this metric?
- TIME-BOUND: Can the department significantly influence the metric in the given time frame?
- DIFFICULT TO GAME: Does the metric eliminate “perverse incentives” to avoid true improvement?
- SIMPLIFIED: Is the metric easy to understand and not an amalgamation of many calculations?
While these are framed in terms of department-level metrics, they clearly apply at all levels of a university. And, finally, Wilsdon et al. (2015) refined responsible metrics as having the following dimensions:
- ROBUSTNESS: basing metrics on the best possible data in terms of accuracy and scope;
- HUMILITY: recognizing that quantitative evaluation should support – but not supplant – qualitative, expert assessment;
- TRANSPARENCY: keeping data collection and analytical processes open and transparent, so that those being evaluated can test and verify the results;
- DIVERSITY: accounting for variation by field, and using a range of indicators to reflect and support a plurality of research and researcher career paths across the system;
- REFLEXIVITY: recognizing and anticipating the systemic and potential effects of indicators and updating them in response.
In lieu of adopting the objectives/key results approach, we have proposed the adoption of the EAB and Wilsdon et al. characteristics as the most relevant to academia. In addition, in concert with the measures of data quality in the next section, they largely capture the previous sets of metric characteristics.
DEFINING "QUALITY DATA"
The International Monetary Fund (2003) and the Organisation for Economic Co-operation and Development (OECD) specify seven dimensions of data quality. Here we paraphrase their definitions within the context of strategic planning and other types of organizational performance metrics.
The degree to which the data are useful in a metric for quantifying progress towards a goal or objective.
The degree to which the data, via the metric, correctly estimate or describe the characteristics that they are intended to measure.
The temporal relevance of the data, generally in the sense that the data are available sufficiently quickly so that the resulting metric is of value and may still be acted upon.
The ease with which the data can be obtained, including the ease with which the data can be accessed.
The ease with which the user may understand and properly use the data for the calculation of the metric or metrics.
The degree to which the data are logically connected and mutually consistent so that they can be brought together with other statistical information within the framework of the metrics and over time.
The confidence that users place in the data, where an important aspect is trust in the objectivity of the data.
Other aspects of data quality include the COMPLETENESS of the data, or conversely, the lack of missing values in a dataset. Incomplete data may result in biased metrics, meaning metrics that systematically under- or over-estimate the quantity of interest.
Quality data should not be based on convenience samples, meaning incomplete datasets that are assembled simply because they are easy to collect. For example, SPOT scores based only on those students who choose to submit scores are convenience samples. Data scraped off the web and only from select databases by Academic Analytics are convenience samples. (For additional concerns about Academic Analytics, see the American Association of University Professors March 22, 2016 "Statements on 'Academic Analystics' and Research Metrics").
Instead, metrics using internal data should be based on census sampling, meaning all the data that is available or, in consultation with a statistician, an appropriate sampling scheme. Properly designed, these methods should help ensure that the metric is accurately estimating the characteristics they are intended to measure (per the Accuracy dimension above).
Finally, no dataset is perfectly complete, nor will the resulting metric perfectly measure the characteristic of interest. Thus, a final measure of quality is the extent to which the data and associated metric are transparent about what they do not measure.
KEY PRINCIPLES
This section presents and describes ten key principles for properly defining and applying metrics. They assume that those defining and selecting metrics will take into consideration the previous discussions on what it means for a metric to be “good” as well as what it means for data to be of high quality.
The very first step should always be careful definition of the objective or goal. Only after careful definition of the objective or goal should the metric or metrics be selected.
The most important consideration when selecting the metric or metrics is how well the metric or metrics will characterize progress towards the goal or objective. Thus, it is key that the metric or metrics be selected with the particular goal or objective in mind.
Corollaries:
- Metrics that do not measure progress towards the goal or objective are of no use.
- Selecting metrics in advance of defining the goal or objective to be achieved is potentially a waste of time.
The number of metrics affiliated with any given goal or objective should be kept as small as possible; more is not always better.
One should always select the smallest number of metrics that adequately characterize performance towards the goal or objective. “In general, each objective should be tied to five or fewer key results [i.e., metrics]” (Doerr, 2018, p. 33). Too many metrics make it easy to lose sight of the objective, perhaps to game the system, and to understand what action to take.
Corollaries:
- Complexity is the enemy of understanding. When in doubt, apply the KISS principle: Keep It Simple, Stupid.
- If it’s not possible to characterize performance towards the goal or objective with a reasonably small number of metrics, it may be that the goal or objective is either too complicated or ill-defined.
A metric based on weak or poor data, no matter how well defined and intentioned, should not be used.
The quality of the data upon which a metric is based is critical and it is not possible to have a good metric that is based on poor or weak data. When using proxy measures, because direct measurement is not possible for some reason, it is equally important to base the proxy measurements on good data. Most importantly, the notion that a metric based on poor data will lead to good decision making is simply wishful thinking.
Corollaries:
- Just as we require rigorous data collection leading to good data in our academic research, so we should require equally good data practices in the management of our academic enterprise.
- It is easier to collect good data on our own operations and internal processes than on external processes or entities.
When using metrics to compare between two or more organizations, the data upon which the metrics are calculated must be equivalent between the organizations.
This is nothing more than common sense for avoiding apples-to-oranges comparisons. It is possible that two different sets of data will be highly correlated, and thus it may be possible to at least compare trends over time between organizations, but without equivalent data no direct performance comparisons can be made.
Corollaries:
- This means that in general it will be difficult at best, and likely impossible, to compare metrics based on internal data with external entities since the equivalent data for the external entities is unlikely to be available.
The cost of calculating the metric, either in terms of dollars and/or time, should be taken into consideration. All things be equal or nearly equal, the metric that costs less or that can be calculated quicker or easier should be preferred.
As the section on Defining a Good Metric discussed, metrics should be relatively simple to measure and thus be both inexpensive and quick to calculate, again relatively speaking. For metrics based on internal data, the costs may be in terms of staff time to compile the data if it is already routinely collected.
Corollaries:
- If the data are not already being collected, then the cost in terms of dollars and/or time will likely be significant.
Metrics should be defined in the appropriate units and with the proper denominator (in the case of a ratio) so that they reflect the desired organizational performance and do not confound that performance with exogenous factors.
Corollaries:
- Financial data displayed as trends over time must be presented in constant dollars. Using real dollars confounds the effect of inflation with actual performance and should be avoided. For example, showing growth over time without adjusting for inflation overstates the actual growth.
- Metrics that are a function of organizational size or some aspect of size should be reported on a per capita basis. For example, reporting the number of SCHs delivered should be per capita because changes in total SCHs will be confounded with changes in faculty size.
Metrics should be crafted to minimize the tendencies toward perverse incentives. This means metrics should always be subjected to anticipatory analysis to discern likely problems that might emerge as a manifestation of perverse incentivization.
As discussed in Edwards and Roy (2016, see Table I), well-intended metrics can result in perverse incentives. Thus, to the extent possible, metrics should be chosen or crafted that minimize these perverse incentives.
Metrics for assessing research and scholarship must follow the ten principles of The Leiden Manifesto.
The Leiden Manifesto was written as a “distillation of best practice in metrics-based research assessment so that researchers can hold evaluators to account, and evaluators can hold their indicators to account” (Hicks et al, 2015, p. 430). These practices should be applied in all aspects of university operations that use metrics to assess research and scholarship, including the Partnership for Incentive-Based Budget as well as promotion and tenure.
The Leiden Manifesto specifies the following ten principles:
- Quantitative evaluation should support qualitative, expert assessment.
- Measure performance should be against the research missions of the institution, group, or researcher.
- Protect excellence in locally relevant research.
- Keep data collection and analytical processes open, transparent and simple.
- Allow those evaluated to verify data and analysis.
- Account for variation by field in publication and citation practices.
- Base assessment of individual researchers on a qualitative judgment of their portfolio.
- Avoid misplaced concreteness and false precision.
- Recognize the systemic effects of assessment and indicators.
- Scrutinize indicators regularly and update them.
Of course, many of these also apply to the use of metrics for other purposes, including strategic planning, particularly items 2, 4, 5 and 8-10. Indeed, had these six not been included in The Leiden Manifesto, then this section would have consisted of 15 principles.
The process of measurement should not influence the objects being measured, else the measurement is made less valid. (Muller, 2018, p. 177).
Goodhart’s Law states that ‘‘when a measure becomes a target, it ceases to be a good measure’’ which means that systems will tend to optimize performance in terms of the metrics, often in spite of the consequences (Koehrsen, 2018). This effect can be particularly pernicious when the metric or measurement is tied to funding, but it can also arise in teaching evaluation scores and other systems where the object of measurement is a person’s or organization’s performance.
Corollary:
- If the goal of a metric or metrics is to influence performance, then significant care must be taken to avoid negative outcomes, including perverse incentivization (see Principle #7), “metric fixation,” and “short-termism” (see Muller, 2018b).
“Not everything that can be counted counts, and not everything that counts can be counted” (Cameron, 1963, p. 13).
We conclude with this principle to underscore the point that reducing complex issues/objectives to summary metrics may not always the best strategy. In particular, metrics are not a substitute for management and, particularly when assessing performance, qualitative information can be critically important for understanding and putting the quantitative metrics results in an appropriate context.
DISCUSSION: METRICS AND THE STRATEGIC PLAN
The definition, application, and use of metrics in our strategic planning process should also be consistent with the following points.
- Strategic planning is a continual process and should be approached as such by scheduling periodic review of active objectives and implementation. As time passes and new opportunities emerge, it will become essential to adjust for these developments.
- The strategic planning should distinguish between metrics for assessment and metrics for incentivization, particularly incentive metrics in the Partnership for Incentive-Based Budget model. In addition, strategic planning should distinguish between stretch/aspirational goals and actual/essential goals.
- Metrics should support the key objectives of the strategic planning process while simultaneously being consistent with and supportive of the strategic plan’s core values. In no case, should a metric contradict a core value or incentivize behavior that would violate a core value.
- Metrics should be aligned between all levels of the organization. In particular, metrics for the Partnership for Incentive-Based Budget should flow from and support the objectives and core values of the strategic plan.
- Metrics should be conscious of the broader context and work in concert to address negative externalities and secondary effects that undermine other objectives. For instance, a metric basis that incentivizes offering large-enrollment courses will need to be paired with another metric that incentivizes teaching small courses that privilege experiential learning and seminar-style engagement.
- Per the Leiden Manifesto, metrics should be based on the plurality of ways that excellence is manifested across multiples colleges and disciplines throughout the university. For instance, research metrics in STEM disciplines should not be applied as a universal norm across the entire university (Alliance for the Arts in Research University, 2018). Disciplinary norms for research or creative discovery in performing arts or literary fields should be applied with equal sensitivity as that associated with traditional norms in STEM fields.
- Metrics should be designed for iteration over the long-term so that the institution can recalibrate progress toward metrics over the long-term. Examples include periodically assessing fundraising/advancement progress and recalibrating the targets for enrollment growth.
- Core values and strategic objectives should drive the balance between internal and external factors for metrics.
- Iterative, continuous assessment with feedback is essential to successful continual planning. In the context of metrics, it is important to create constructive ways to retool objective and metrics when targets are missed.
American Association of University Professors (2016). “Statement on ‘Academic Analytics’ and Research Metrics” dated March 22, 2016. Accessed online at www.aaup.org/file/ AcademicAnalytics_statement.pdfon June 9, 2018.
Alliance for the Arts in Research Universities. (2018). What is Research? Practices in the arts, research, and curricula. Ann Arbor, MI: University of Michigan.
Benedictus, R., and F. Miedema (2016). Fewer Numbers, Better Science, Nature, 538, 453-455.
Cameron, W.B. (1963). Informal Sociology: A Casual Introduction to Sociological Thinking, Random House, New York, NY.
Carpenter, C.R., Cone, D.C., and C.C. Sarli (2014). Using Publication Metrics to Highlight Academic Productivity and Research Impact, Academic Emergency Medicine, 21, 1160-1172.
Doerr, J. (2018). Measure What Matters, Portfolio/Penguin, New York, NY.
EAB (2018). Academic Vital Signs: Aligning Departmental Evaluation with Institutional Priorities. Accessed online at https://www.eab.com/research-and-insights/academic-affairs- forum/studies/2018/academic-vital-signson June 12, 2018.
Edwards, M.A., and S. Roy (2017). Academic Research in the 21st Century: Maintaining Scientific Integrity in a Climate of Perverse Incentives and Hypercompetition, Environmental Engineering Science, 34, 51-61.
Ferguson, M.W.J. (2016). Treat Metrics Only as Surrogates, Nature, 538, 455.
Godfrey, A.B. (2008) Eye on Data Quality, Six Sigma Forum Magazine, 8, 5–6.
Hicks, D., P. Wouters, L. Waltman, S. de Rijcke, and I. Rofols (2015). The Leiden Manifesto for Research Metrics, Nature,250, 429-431.
IMF (2003). Data Quality Assessment Framework (DQAF), Chapter IX. Data Quality and Metadata, accessed online at https://unstats.un.org/oslogroup/meetings/ og-04/docs/oslo-group-meeting-04--escm-ch09-draft1.pdfon June 5, 2018.
Ioannidis, J.P.A., and M.J. Khoury (2014). Assessing Value in Biomedical Research: The PQRST of Appraisal and Reward, Journal of the American Medical Association, 312, 483-484
Jump, P. (2015), “Metrics: How to Handle Them Responsibly,” Times Higher Education. Accessed online at www.timeshighereducation.com/features/metrics-how-to- handle-them-responsiblyon June 9, 2018.
Koehrsen, W. (2018). Unintended Consequences and Goodhart’s Law: The importance of using the right metrics, dated February 24. Accessed online at https://towardsdatascience. com/unintended-consequences-and-goodharts-law-68d60a94705con June 5, 2018.
McNutt, M. (2014). The Measure of Research Merit, Nature, 346, 1155.
Merriam-Webster (2018). Definition of Metric, updated on 27 May 2018. Accessed at www.merriam-webster.com/dictionary/metricon June 4, 2018.
Moher, D., Naudet, F., Cristea, I.A., Miedema, F., Ioannidis, J.P.A., and S.N. Goodman (2018). Assessing Scientists for Hiring, Promotion, and Tenure, PLOS Biology, https://doi.org/10.1371/journal.pbio.2004089.
Muller, J.Z. (2018a). The Tyranny of Metrics, Princeton University Press, Princeton, NJ.
Muller, J.Z. (2018b). Against Metrics: How Measuring Performance by Numbers Backfires, Aeon. Accessed online at https://aeon.co/ideas/against-metrics- how-measuring-performance-by-numbers-backfireson June 5, 2018.
OECD (2011). Quality Dimensions, Core Values for OECD Statistics and Procedures for Planning and Evaluation Statistical Activities, STD/QFS(2011)1, accessed online at www.oecd.org/sdd/21687665.pdfon June 5, 2018.
Oxford Living Dictionary (2018). Main Definitions of Metric in English. Accessed at http://en.oxforddictionaries.com/definition/metricon June 4, 2018.
Tremmell, J. (2016). The 5 Characteristics of an Effective Business Metric, Inc., September 9. Accessed online at www.inc.com/joel-trammell/the-5- characteristics-of-an-effective-business-metric.htmlon June 4, 2018.
TDWI Blog (2010). 12 Characteristics of Effective Metrics, April 19. Accessed online at https://tdwi.org/Blogs/TDWI-Blog/2010/04/Effective-Metrics.aspxon June 4, 2018.
Wilsdon, J. et al. (2015). The Metric Tide: Report of the Independent Review of the Role of Metrics in Research Assessment and Management. DOI: 10.13140/RG.2.1.4929.1363
Yoskovitz, B.. (2013). Measuring What Matters: How To Pick A Good Metric, OnStartups.com, March 29. Accessed online at www.onstartups.com/tabid/ 3339/bid/96738/Measuring-What-Matters-How-To-Pick-A-Good-Metric.aspxon June 4, 2018.
RANKINGS WHITE PAPER
A TOP NATIONALLY AND GLOBALLY RECOGNIZED UNIVERSITY
Virginia Tech aspires to be a top nationally and globally recognized public land-grant university. Specifically, our goal is to be a member of that rarified set of universities that are recognized nationally and globally for their excellence in research and education, for their superiority in creativity and innovation, and for their worldwide outreach and service.
Evaluating our progress towards becoming a top recognized public land-grant university will be partially based on various rankings such as the Times Higher Education (THE) World University Rankings and Wall Street Journal/Times Higher Education (WSJ/THE) U.S. College Rankings. However, we recognize that these rankings are, at best, proxy measures that neither fully reflect our unique aspirations as a university nor all the relevant and important dimensions of our reputation.
That said, we also recognize that each of the university ranking schemes captures some important aspects of a university’s performance. We further recognize that, broadly speaking, both tangible and intangible benefits accrue to universities that are highly ranked. For example, global reputation is important for both international partnerships, collaborations, and enrollments. Similarly, prestigious international institutions, governments, and corporations are increasingly considering global rankings as they look for the institutions, academic programs, and faculty with whom they would like to partner. Furthermore, qualified international students look to rankings in making their enrollment decisions.
However, while we will use the various university rankings as one way to assess our progress towards becoming a nationally and globally recognized top public land-grant university, Virginia Tech will not change who we are to match or optimize our performance in the rankings. We are proud of who we are, particularly of our land-grant heritage, and we seek to bring that reputation to the world.
In this Strategic Plan, we take the point of view that tracking and managing metrics related to university rankings need not come at the expense of compromising Virginia Tech’s values and core identity, particularly Ut Prosim (That I May Serve). The key idea is not to pursue rankings at the expense of our identity – it is to improve our ranking while maintaining our unique identity.We will accurately reflect university activities to improve our standing in the various rankings, and we will align activities and practices within university operations to maximally support and promote our research and creative enterprise, and we will do so without compromising our principles or our unique identity as a university.
TIMES HIGHER EDUCATION WORLD UNIVERSITY RANKINGS AND WALL STREET JOURNAL/ TIMES HIGHER EDUCATION U.S. COLLEGE RANKINGS, IN BRIEF
Rankings are based on a variety of measures, all quantified and weighted differently by the various ranking schemes. The charts below summarize the measures used by the two ranking organizations previously mentioned:
WALL STREET JOURNAL/TIMES HIGHER EDUCATION U.S. COLLEGE RANKINGS (WSJ/THE)
Learning Environment | 73% |
Reputation | 17% |
Research | 8% |
Internationalization | 2% |
Figure 1. Wall Street Journal/Times Higher Education U.S. College Rankings Methodology
TIMES HIGHER EDUCATION WORLD UNIVERSITY RANKINGS (THE WUR)
Reputation | 33% |
Citations | 30% |
Research | 20% |
Internationalization | 8% |
Income | 5% |
Learning Environment | 4% |
Figure 2. Times Higher Education World University Rankings Methodology
As the charts show, Wall Street Journal/Times Higher Education U.S. College Rankings is undergraduate and teaching oriented, focusing on the following pillars: outcomes, resources, engagement, and environment. While Times Higher Education World University Rankings is a more comprehensive ranking scheme that is research oriented, focusing on the following pillars: teaching, research, citations, industry income, international outlook. When considered collectively, these rankings provide a comprehensive view of the of the land-grant mission on both a national and global scale.
There are two common factors that cut across both ranking systems. They are:
- Reputation measures based on surveys of academics and students to gather their subjective judgements;
- Research measures, including citation measures, based on data collected from the institution and Elsevier’s Scopus, a database of peer-reviewed literature.
Closely related to, and indeed underlying the citations measures, are publications, where quality and high impact publications typically and ideally drive the citation rates. Other factors that contribute to a global reputation include:
- National and international visibility, including faculty participation, particularly speaking at international conferences and other events;
- An effective communications strategy that raises the visibility of the institution in a variety of national and international media, including both traditional media and emerging new forms of media;
- And an effective strategy for engaging and leveraging alumni networks.
A naive approach to improving a university’s reputation, particularly as measured by the number of publications and the citation rate, would be to encourage and/or incentivize the faculty to increase their output as measured by these metrics. This strategic plan explicitly rejects this approach for the following two reasons. First, simply encouraging faculty to increase publication output and/or citation rates is an exercise fraught with perverse incentives. The objective of research conducted at Virginia Tech is impactful, high quality scholarship; publication output and citation rates are but proxy measures for this type of activity, not the end goals. Second, incentivizing publication and/or citation rates may yield short-term improvements, but it will not likely result in sustained output. Quality scholarly publications and high citation rates are output measures of a faculty engaged in impactful research. The inputs are the critical drivers of long-term success: recruitment and retention of a world class faculty supported by systems, processes, and resources that facilitate the conduct of high-quality research.
NEXT STEPS
The university has set the following milestones within the strategic planning framework: Virginia Tech will be a top 10 U.S. public land-grant according to Wall Street Journal/Times Higher Education U.S. College Rankings and a top 13 U.S. public land-grant according to Times Higher Education World University Rankings by 2024. Immediate steps that Virginia Tech will take, indeed is already taking, to make sure that the university’s current performance is appropriately and properly reflected in the rankings include:
- Ensuring the various rankings organizations are capturing all the university’s scholarly and creative activities and publications.
- Similarly, ensuring that those databases used to quantify citation counts, such as Scopus, are fully utilized so that all citations are captured. For example, ensuring that faculty have the necessary access and training to access Scopus so that they may correct any errors in their data.
- Significantly increasing placements in both national and international media that promote the university and increase Virginia Tech’s research profile. Similarly, redoubling efforts to increase brand recognition more broadly worldwide.
- Undertaking a study of those typically surveyed by the ranking organizations to understand how Virginia Tech is perceived in terms of scholarship and other measures contributing to our national and international reputation. Implement appropriate measures to address any shortcomings.
To substantially improve the university’s national and international reputation, in order for Virginia Tech to meet the above milestones, requires significant and sustained investment in faculty and infrastructure.
PARTNERSHIP FOR AN INCENTIVE-BASED BUDGET (PIBB) MODEL
Beyond Boundaries imagines a university with greater financial resilience, funded by a diverse resource base and supported by budget models that enable adaptability and innovation in an increasingly dynamic academic environment and shifting financial landscape. The Partnership for Incentive-Based Budget model is one of the new funding models established within the university to realize this vision.
GUIDING PRINCIPLES
As the main funding model for the university’s academic programs, the Partnership for Incentive-Based Budget model is intended to integrate university strategic planning with the budget process to ensure that resources are allocated in a manner that supports the university’s core mission and vision. Primary principles guiding the development of the Partnership for Incentive-Based Budget model are as follows:
- The budget model must connect resource allocations to accomplishing objectives of university strategic plan
- The budget model should promote growth and diversification of university resources
- The budget model should reward performance outputs and outcomes that are relevant, clearly defined, and easily measured
- The set of chosen performance metrics should reflect both shared and distinctive strategic outcomes expected from a comprehensive university
- Performance goals and milestones should be established in collaboration with units being assessed
- The budget model should encourage and reward inter- and transdisciplinary instruction, research, and outreach
- The budget model should promote institutional decision-making based on valid data accessible to units being assessed
- The budget model and associated budget development processes must ensure transparency in resource decision-making
- The budget model must foster the ability to conduct long-range planning
- The budget model must enable the university to manage resources effectively in a dynamic academic and financial environment
- The budget model must enable adjustments to resource allocations based on actual performance
STRUCTURE OF THE PARTNERSHIP FOR INCENTIVE-BASED BUDGET MODEL
The Office of the Executive Vice President and Provost continues to work with degree-granting colleges to develop a model that sufficiently resources the academic enterprise, while incenting activities in strategically important directions. To accomplish this, the Partnership for Incentive-Based Budget model has been structured around three major budget components that are combined to calculate the overall budget for academic areas as shown in Figure 1: Unit Allocations, Scorecard Allocations, and Earmarked Allocations. The Unit Allocations and Scorecard Allocations are part of a formulaic distribution of resources based on the achievement of annually established milestones across a broad range of performance metrics. Earmarked Allocations are funding reserved to support specific activities in certain areas of the university.
The major budget components of the Partnership for Incentive-Based Budget Model that equal the ANNUAL COLLEGE BUDGET are UNIT ALLOCATIONS plus SCORECARD ALLOCATIONS plus EARMARKED ALLOCATIONS.
Unit Allocations: Based on metrics to incent revenue growth and diversification, and strategic priorities of the university.
Student Credit Hours (SCHs)
SCH Premiums:
- SCHs to Other College Majors
- SCHs in Target Section Sizes
- SCHs in Honors Courses
- SCHs in Pathway Courses
Enrollments
Enrollment Premiums:
- Out-of-State Majors
- Students in More Than 1 Major
New Gifts & Commitments
New Gifts & Commitment Premiums:
- Scholarship Endowments
- Professorship Endowments
Sponsored Expenditures
Ancillary Income
- PLUS -
Scorecard Allocations: Based on metrics to incent broader university outcomes, strategic priorities of the university.
Faculty Success Metrics:
- Faculty Diversity
- TBD*
Student Success Metrics:
- Student Diversity
- 4-Year Graduate Rate URM/USS Disparities for Entering Freshmen3-Year Graduation Rate URM/USS Disparities for Transfer Students
- TBD*
Administrative Effectiveness Metrics:
- TBD*
*Fiscal Year 2019-20 is the first year for piloting Scorecard metrics. Further metrics will be incorporated in later years
- PLUS -
Earmarked Allocations: Funding reserved to support more specific university activities, and strategic priorities of the university.
Ancillary Income
MD/VA DVM Capitation Allocation
College Program Fees
Special Sessions Revenue Share
VT/Carilion School of Medical Allocation
Enterprise Programs Revenue Share
Equipment Trust Funds
Destination Area Faculty Allocation
Self-Supporting Degrees Revenue Share
Course Fees
Metrics associated with Unit Allocations of the Partnership for Incentive-Based Budget model are primarily intended to incentivize growth in major revenue generating activities of the institution. These include, student credit hours and enrollments to reflect the institution’s increased reliance on tuition to support educational costs. Also reflected in this portion of the budget model are metrics associated with growing the external funding that the university receives to support operations, including new gifts and commitments provided through fundraising, extramural grant and contract funding for sponsored expenditures, and ancillary income generated from university sales and services. These metrics are termed “unit allocation” metrics because they are assigned a unit of value for each unit of output. For example, in Fiscal Year 2018-19, the Partnership for Incentive-Based Budget model allocated $107.75 per student credit hour to colleges as part of the Unit Allocations budget component.
Some Unit Allocation metrics have an additional budget value, or premium, attached to a subset of the metric’s output in order to incentivize strategically important activities that go beyond revenue generation. For example, in Fiscal Year 2018-19 student credit hours delivered to students whose majors were outside of the instructing college received a premium of $10.00 per student credit hour to incentivize interdisciplinary instruction. In this example, this $10.00 premium is added to the baseline student credit hour value of $107.75, increasing the per unit budget value to $117.75 per student credit hour. Similar premiums are or will be provided for courses within targeted class sizes, honors courses, Pathways to General Education courses, courses supporting the university’s Destination Areas initiative, out-of-state enrollments, students enrolled in more than one major, industry-funded sponsored expenditures, and new gifts and commitments that support scholarship or professorship endowments. Additional premiums may be developed over time to incentivize activities that support the university’s strategic plan.
In the Unit Allocation portion of the Partnership for Incentive-Based Budget model long-range goals and short-term milestones are established for each metric annually in consultation with the colleges and university administration to ensure that the university’s infrastructure can accommodate projected enrollments, that sufficient instructional resources can be deployed to teach projected class loads, and that external funding targets are attainable. The goal-based nature of the Partnership for Incentive-Based Budget model and its intentional connections to university strategic priorities differentiates it from the pure revenue-sharing budget models currently established at many peer institutions.
Another characteristic that distinguishes the Partnership for Incentive-Based Budget model from other university budget models is its reliance on a broader array of outcomes and activities expected from a comprehensive university. The metrics in the Scorecard Allocation portion of the Partnership for Incentive-Based Budget model captures these outcomes in three broad categories: faculty success, student success, and administrative effectiveness.
The Scorecard will include summarized measures of faculty activity and faculty composition. These metrics will be drawn from teaching data, faculty activity reporting systems, and other sources to include broad categories like faculty teaching, scholarship, engagement, and diversity.
The Scorecard will also include a broad range of commonly understood student outcomes. These will include admissions process metrics, progress s to degree metrics, outcomes for graduating students, and student participation in the broad range of curricular and extracurricular experiences that promote the “VT-shaped” student goals of the Strategic Plan. It will also include measures of student diversity and the opportunity to look at a broad range of outcomes for various populations of underrepresented and underserved students.
A third area of metrics will be related to administrative effectiveness. These metrics will monitor institutional efforts in continuous improvement and compliance with important external regulatory requirements.
The Scorecard portion of the budget is not a metric-by-metric calculation but rather treated as a block grant with a portion subject to a review and allotment process. Annually, the Provost, the College Dean and related Vice Provosts or Vice Presidents will jointly review Scorecard goals and achievements toward those goals. When progress towards expected outcomes in a Scorecard Metric is not made, a cooperative, qualitative evaluation of the activity will be undertaken and funds from the college, academic administration and, where appropriate, central resources will be applied to a jointly developed plan addressing outcomes in the particular area.
Fiscal Year 2019-20 will be the first year that detailed Scorecard metrics are incorporated in the Partnership for Incentive-Based Budget. The first Scorecard metrics will focus on gender and racial diversity among tenured/tenured track and non-tenured instructional faculty, the 4-year graduation rates for students who enter the university as freshmen, the 3-year graduation rates for students who enter the university as transfers, and disparities in the graduation rates for underrepresented minority and underserved students.
The third component of the Partnership for Incentive-Based Budget model is Earmarked Allocations that support specific university activities. These include program and course fees allocated directly to colleges to cover extraordinary costs of instruction associated with some degrees and courses; special session revenues for courses taught during winter and summer terms that are shared with the colleges (approximately 70% returned to the college and 30% retained centrally); self-supporting, professionally oriented programs that are anticipated to charge a market rate of tuition and enroll sufficient students to generate net income that will enhance the resources for the college, department and the institution; external income from ancillary operations who charge for services that also support their instructional activities (e.g., the Veterinary Teaching Hospital, the Adult Day Care Center, the Child Development Center); and other college-specific allocations in support of strategic academic programs and initiatives.
NEXT STEPS
The Office of the Executive Vice President and Provost will continue work with the colleges and the university’s administration to refine and, as appropriate, develop new metrics that support the strategic goals of the university. In parallel with this effort, the university is continuously improving the information systems necessary to support the new budget model and other associated strategic decision-making processes and structures (e.g., undergraduate enrollment management, faculty activity reporting, graduate program management, strategic planning metric tracking, and other ad hoc analyses).